Data For Social Good (D4SG) Project: AutoCut Onboarding Flow
The Data For Social Good (D4SG) project focused on addressing real-world challenges faced by political directors when managing canvassing territories. Working alongside a nonprofit organization, our team developed the AutoCut feature, a powerful tool designed to automate and optimize the division of geographical areas, reducing time and potential errors.
As part of this initiative, my key responsibility was to design an intuitive onboarding experience that allowed users to seamlessly integrate this advanced tool into their daily workflow. While the AutoCut feature automated much of the process, careful attention to user experience and education was required to ensure political directors could adopt the system with ease and confidence.
This project provided valuable hands-on experience in solving real-world problems through design and collaboration with stakeholders outside of the classroom, further enhancing my understanding of UX in applied settings.
Wireframes & User Flow
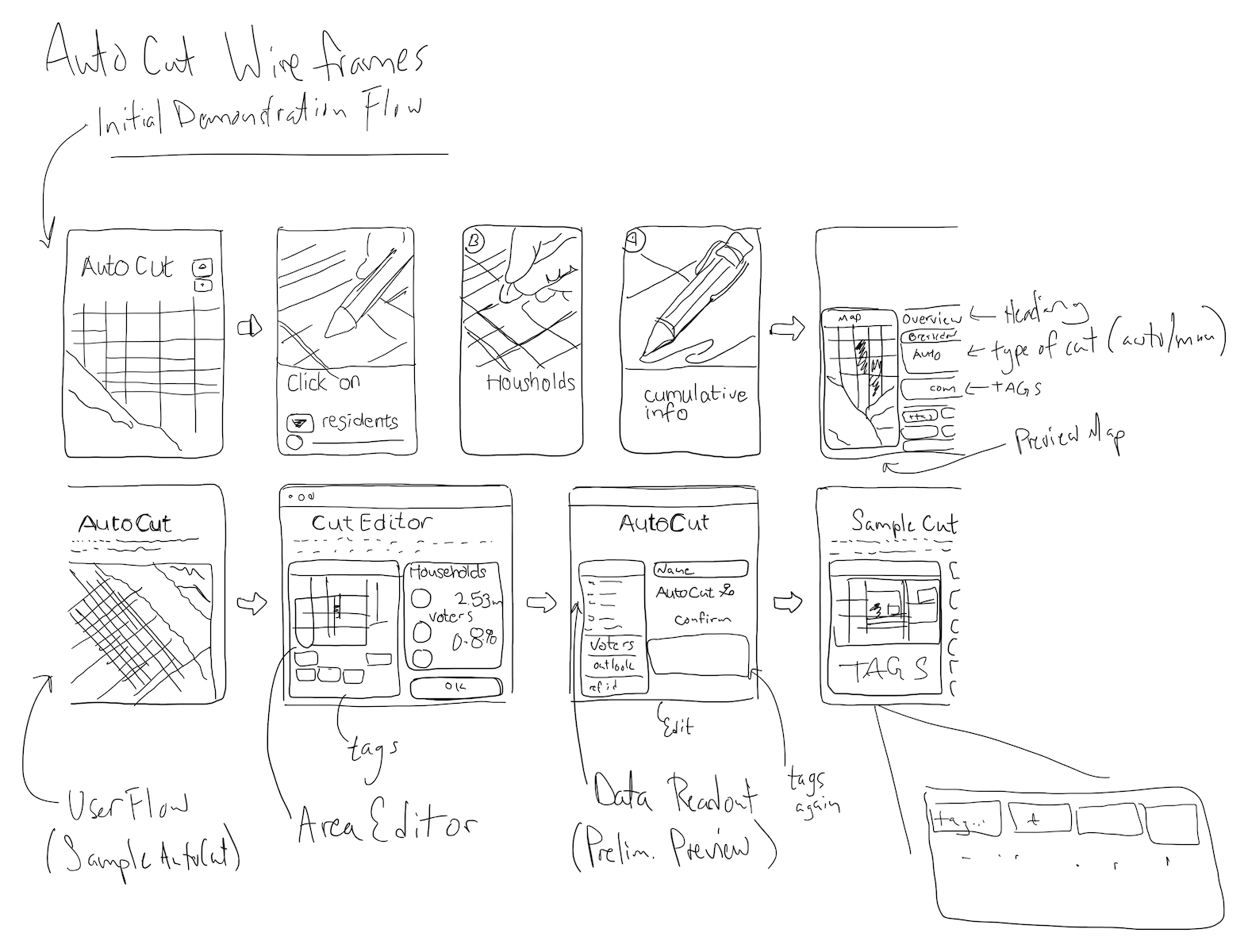
The wireframes illustrate the step-by-step onboarding flow we designed for AutoCut. The goal was to simplify the process while reducing the cognitive load on political directors. The flow automates turf-cutting based on predefined metrics such as population size, offering a streamlined user experience that helps directors focus on high-level decision-making rather than manual setup.
Design Decisions
The most significant challenge in designing this feature was balancing automation with manual control. Political directors needed enough flexibility to make decisions but appreciated the convenience of automated suggestions. We focused on three primary elements to achieve this:
- Automated Suggestions: Based on user feedback, we integrated automated suggestions for turf divisions, allowing directors to simply confirm or adjust pre-generated cuts rather than start from scratch.
- Clear Feedback: Providing real-time feedback on actions, such as success markers and warnings, was crucial in minimizing errors and increasing confidence in the system.
- Progress Indicators: By including visual progress indicators, users were able to understand where they were in the process, reducing confusion and frustration.
Key Insights & Learnings
This project revealed several important insights that helped shape the final design:
- User Education: During early user testing, it became clear that some terminology used within the platform was not intuitive for all users. Terms like "Turf" and "Universe" were unfamiliar to many political directors. To resolve this, we introduced tooltips and a simplified glossary that explained these terms within the context of the system.
- Automation Preference: Users preferred the ease of automated turf suggestions, as it reduced their workload significantly. This led to refining the AutoCut feature to provide users with optimal cuts based on the number of households and other metrics.
- Progress Visibility: Feedback indicated that users wanted to see how far they were in the turf-cutting process. Step-by-step progress indicators were added to ensure that users felt in control and knew when tasks were completed.
- Clear Feedback: Visual feedback, such as immediate success markers when actions were completed correctly, played a critical role in reducing errors and building trust in the system. This feature was particularly appreciated by users who felt overwhelmed by the technical aspects of turf-cutting.
Onboarding Flow Process
The onboarding process introduced new users to the AutoCut feature step-by-step. Each phase of the process was designed with clear instructions, contextual help, and visual cues. Key design elements like progress bars, tooltips, and guided prompts were implemented to ensure users had the guidance they needed without being overwhelmed. This design allowed political directors to transition seamlessly into using AutoCut without any prior experience with similar tools.
Final Design
The final design integrated all the feedback we gathered during testing. By focusing on reducing cognitive load, offering clear visual feedback, and guiding users through the process with contextual help, we ensured that even users unfamiliar with technology could confidently and effectively use the AutoCut tool. The streamlined onboarding experience was a key factor in improving user engagement and overall satisfaction.
Reflection & Future Considerations
While the AutoCut onboarding flow significantly improved user engagement, there’s always room for iteration and growth. Balancing automation with manual control was one of the key challenges, and while the feedback was positive, a few areas stand out for potential improvements.
- Deeper Personalization: Future versions could integrate more granular control over turf-cutting suggestions. Machine learning could adapt the suggestions based on users’ past preferences or campaign goals, giving users more trust in the system as it evolves with their needs.
- Improved Visual Feedback: Expanding the visual feedback system with micro-interactions and dynamic color shifts could further enhance clarity and provide users with real-time assurance, reducing friction in more complex tasks.
- Enhanced Collaboration Features: Future iterations could benefit from team collaboration features such as shared suggestions, comments, and real-time editing. This would align with the trend of making digital tools more inclusive and collaborative.
Reflection: This project taught me the importance of balancing simplicity with power. It was rewarding to see the positive impact AutoCut had on political directors' workflows, yet I recognize that the design is never truly finished. There’s always more to learn and adapt. Moving forward, I’m excited by the potential for more AI-driven personalization and collaborative features. Ultimately, this project reaffirmed my belief that design is a constant journey of refinement and learning, rather than a final destination.